Revolutionizing Warehouse Robotics - Inside Dexterity's Platform
Dexterity has pioneered using one robot AI platform that can power the most complicated applications in the warehouse.


Author: Varun Nayak, Senior Robotics Engineer
Think about all the warehouse tasks that deal with a dynamic, unknown, complex variety of goods. You might think about palletization, depalletization, order fulfillment, induction, and truck loading—all these tasks require a person to react to the task they’re being asked to accomplish.
However, what typically takes a person a few hours of training to learn is challenging for robots. Most traditional robots need months and months of programming or upstream infrastructure changes to make them capable of learning something new.
Until now.
Dexterity has pioneered using one robot AI platform that can power the most complicated applications in the warehouse. Using a combination of cutting-edge and tried-and-true AI approaches, we power robots with the ability to sense, think, and move as a person would in tackling tasks ranging from inbound to outbound.
This means that Dexterity-powered robots can take a complex task like mixed SKU palletizing and reason through the problem like a person would - i.e., ‘I need to pick from here and put over there in a way that meets workflow requirements of stability, order processing, speed, etc.’ Warehouse facilities require this dynamic understanding of complex tasks.
Sensing is Understanding
The foundation of Dexterity’s AI platform is our robust vision system, built using state-of-the-art machine learning models to enable object detection, tracking, and semantic understanding of the environment for reasoning about and operating safely within it.
That is a fancy way of saying that we can look at a broad mix of boxes and understand what a box is, its shape, and the best way to grab it. In the case of palletizing and depalletizing, this capability is used to figure out which box the robot should pick first, either from the pallet or the conveyor. Even if the box is oddly shaped, wrapped in plastic, or tilted on the pallet, Dexterity’s AI can figure out how to pick it.
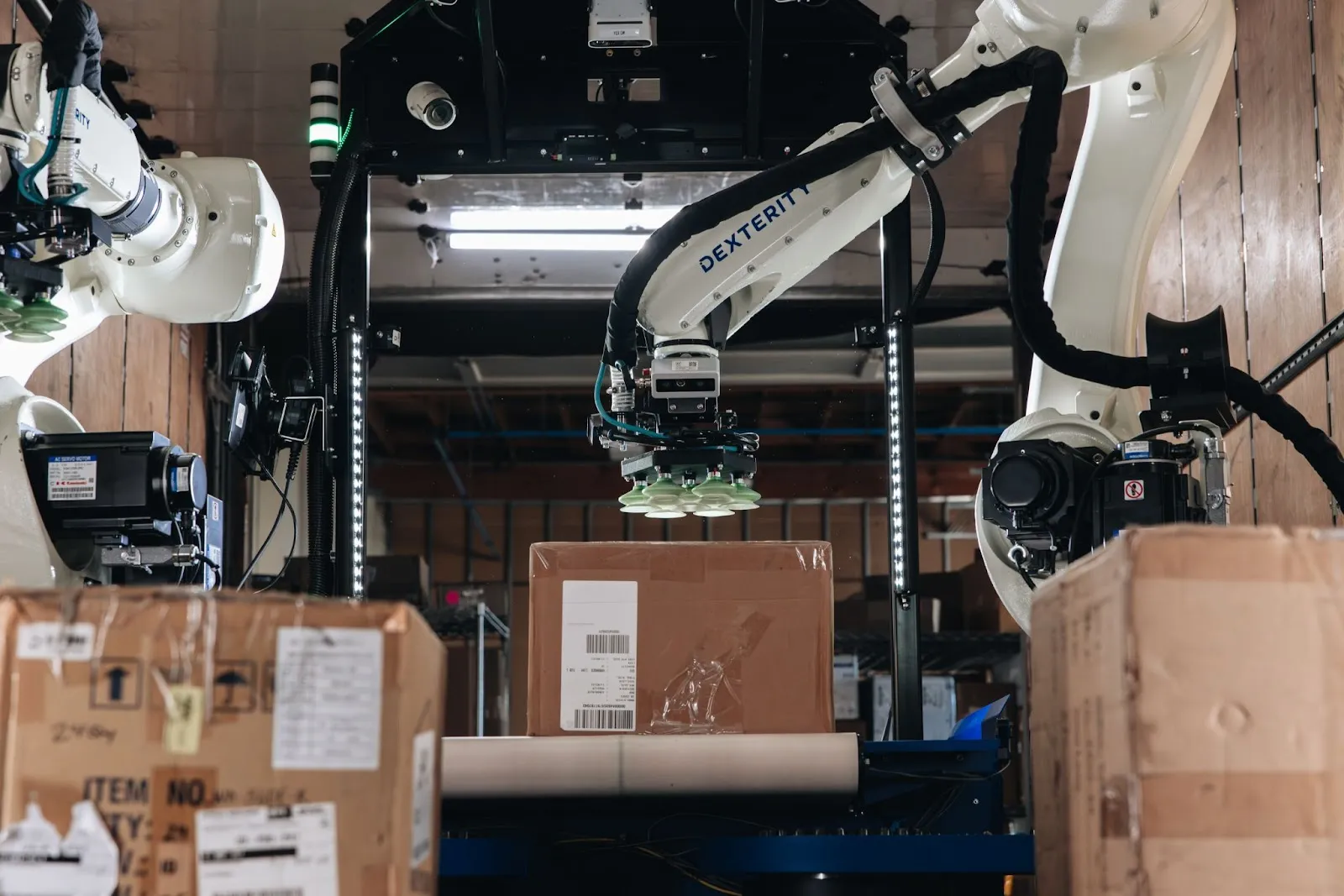
As we pick up the box, we also use force control to figure out how much pressure we can put on an object without damaging it, either when picking it up or putting it down.
This is particularly important when trying to build a stable pallet. You need robots to push boxes together to achieve density on the pallet without pushing boxes to the floor.
Finally, we integrate with external sensors that give the robot additional information about the box it is picking. Weight sensors help understand whether the box is heavy or light. Bar code scanners can indicate whether a package is on pallet #1 or #2. All of this information then feeds into Dexterity’s decision engine.
Decisions, Decisions, Decisions
After we sense the package, box, bag, or tote, we must figure out what to do with it. In the case of depalletizing, that decision can be reasonably straightforward - which of these boxes can I pick first without destabilizing the rest of the pallet?

Packing a pallet, however, is another story. Using a combination of algorithmic planning and machine learning, we can simulate thousands of possibilities for how a box placed on a pallet will impact the stability of the pallet and the crush-ability of the lighter boxes on the pallet.
Dexterity’s machine learning algorithms are leveraged by robots packing onto a pallet, loading mixed boxes onto a truck, or optimizing tray utilization when packing bread. In each one of these examples, robots use sensing information (size, weight, and ‘squishiness’ of the item) compared against a trained model (e.g., a requirement for chimney-stacked pallets) and learned experiences from production to decide on how to load a package onto a pallet.
From Pallet to Conveyor and Back Again
Lastly, Dexterity’s AI platform uniquely uses proprietary motion control to move robot arms. This enables us to plan, avoid obstacles, operate in tighter spaces, and move in smooth trajectories that increase robot throughput.
We also use motion control to react to new information received by the robot. When depalletizing, for instance, if the robot senses that a package is swinging, it will immediately slow down the arm's speed so the box doesn’t sheer off.
Without integrated motion planning, a robot arm performing a complicated task needs more space to operate, taking up more space in the warehouse and often requiring more integration fees. With our dedicated motion control, Dexterity can power robots that drop into existing workspaces and workflows with minimal site retrofitting.
Steel and Silicon - How Dexterity’s software powers multiple robots.
These capabilities are not limited to any particular robot, sensor, or motor.
One of the cornerstones of Dexterity’s approach is the platform’s ability to abstract hardware complexities, enabling integration with various off-the-shelf components, including sensors, actuators, and diverse robot models from different vendors. A Dexterity proposed industry standard, which we call IRIS (Intelligent Robotics Interface Standard), facilitates this.
This abstraction layer not only liberates robots from the confines of proprietary systems but also fosters an ecosystem where innovation thrives through ease of implementation.
Tying It All Together
Why must these all work together and not be discrete models?
By working together, these components create a cohesive system that mimics human-like intelligence and adaptability, creating the perfect foundation for tackling complex challenges in the warehouse environment. Dexterity’s subsystems constantly talk to each other to maximize the KPIs our clients care about.
For example, the vision system provides rich information to make the best possible packing decisions. If a stack of boxes starts tilting, we update our internal model of the pallet so that any stability concerns can be gracefully addressed during subsequent choices.
Another example, while loading a truck, is deciding whether the robot should grasp a box from the top or the front. This depends on the robot's reach, the gripper's geometry, and where the box is intended to go. It is a complex decision-making problem involving both robot geometry and the physics of suction cups working on cardboard surfaces. Accounting for all these factors allows us to pack a truck wall consistently up to its ceiling.
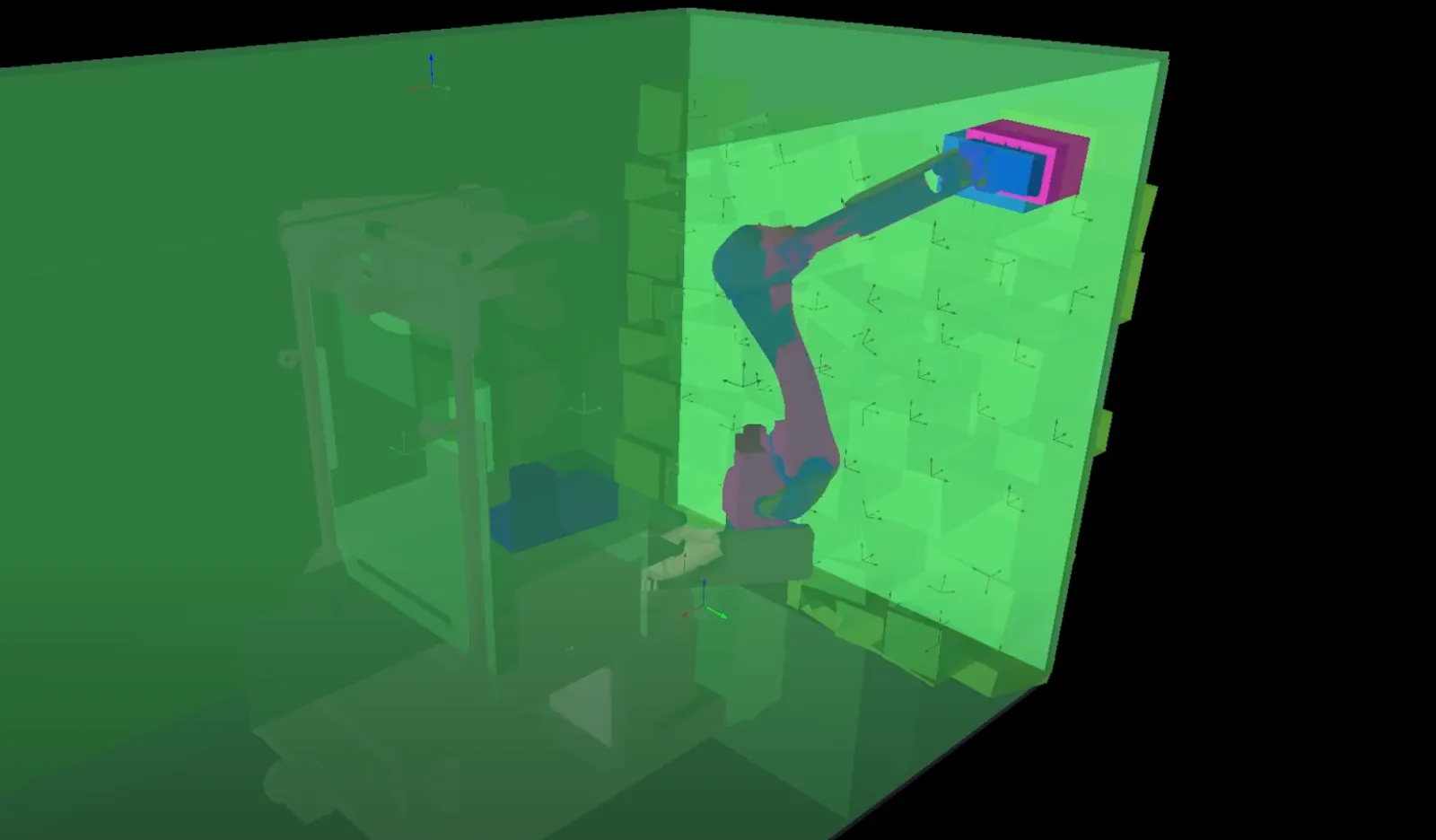
Solving The Entire Problem
In addition to our robotics and AI capabilities, rich observability data and operational metrics from all our systems provide valuable insights into our software and unlock detailed analytics that can improve warehouse operations.
We recognize that customers don’t come to us to purchase a robot. They are seeking a business outcome that is needed in their operations. That is how we combine software, hardware, analytics, and our talented team into outcomes that solve the most challenging warehouse challenges for our customers.
We are moving forward with our robotic truck loading and unloading, mixed-case palletizing and depalletizing, and singulation/induction applications in parcel distribution, third-party logistics providers, retail, and apparel industries. We would love an opportunity to partner with you too! If you would like to explore our applications, we would love the opportunity to learn more about your operations and continue the conversation.